Multimodal Perception and Comprehension of Corner Cases in Autonomous Driving
ECCV 2024 Workshop @ Space 2, MiCo Milano, Italy, Sep 30th Monday
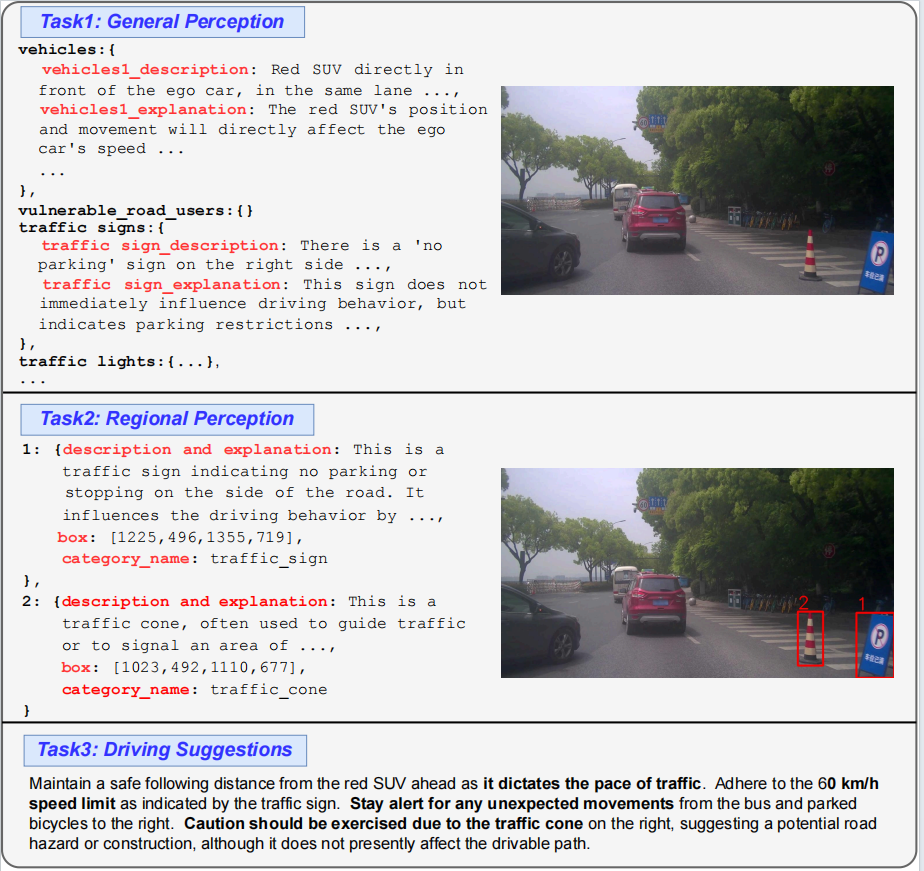
Visual comprehension data samples from CODA-LM.
Introduction
This workshop aims to bridge the gap between state-of-the-art autonomous driving techniques and fully intelligent, reliable self-driving agents, particularly when confronted with corner cases, rare but critical situations that challenge limits of reliable autonomous driving. The advent of Multimodal Large Language Models (MLLMs), represented by GPT-4V, demonstrates the unprecedented capabilities in multimodal perception and comprehension even under dynamic street scenes. However, leveraging MLLMs to tackle the nuanced challenges of self-driving still remains an open field. This workshop seeks to foster innovative research in multimodal perception and comprehension, end-to-end driving systems, and the application of advanced AIGC techniques to autonomous systems. We conduct a challenge comprising two tracks: corner case scene understanding and corner case scene generation. The dual-track challenge is designed to advance reliability and interpretability of autonomous systems in both typical and extreme corner cases.
Challenges
Note: participants are encouraged to submit reports as workshop papers! Check Call for Papers for more details!
Track 1: Corner Case Scene Understanding
This track is designed to enhance perception and comprehension abilities of MLLMs for autonomous driving, focusing on global scene understanding, regional reasoning, and actionable suggestions. With the CODA-LM dataset consisting of 5,000 images with textual descriptions covering global driving scenarios, detailed analyses of corner cases, and future driving recommendation, this track seeks to promote the development of more reliable and interpretable autonomous driving agents.
- Datasets: Please refer to CODA-LM for detailed dataset introduction and dataset downloads.
- Please find more information on the Track1 page.
Track 2: Corner Case Scene Generation
This track focuses on improving the capabilities of diffusion models to create multi-view street scene videos that are consistent with 3D geometric scene descriptors, including the Bird's Eye View (BEV) maps and 3D LiDAR bounding boxes. Building on MagicDrive for controllable 3D video generation, this track aims to advance scene generation for autonomous driving, ensuring better consistency, higher resolution, and longer duration.
- Datasets: Please refer to MagicDrive for detailed dataset introduction and dataset downloads (i.e., nuScenes dataset).
- Please find more information on the Track2 page.
Call for Papers
Overview. This workshop aims to foster innovative research and development in multimodal perception and comprehension of corner cases for autonomous driving, critical for advancing the next-generation industry-level self-driving solutions. Our focus encompasses a broad range of cutting-edge topics, including but not limited to:
- Corner case mining and generation for autonomous driving.
- 3D object detection and scene understanding.
- Semantic occupancy prediction.
- Weakly supervised learning for 3D Lidar and 2D images.
- One/few/zero-shot learning for autonomous perception.
- End-to-end autonomous driving systems with Large Multimodal Models.
- Large Language Models techniques adaptable for self-driving systems.
- Safety/explainability/robustness for end-to-end autonomous driving.
- Domain adaptation and generalization for end-to-end autonomous driving.
Submission tracks. All submissions should be anonymous, and reviewing is double-blind. We encourage two types of submissions:
-
Full workshop papers not previously published or accepted for publication in the substantially
similar form in any peer-reviewed venues including journals, conferences or workshops. Papers are limited
to 14 pages, including both figures and tables, in ECCV format with extra pages containing only cited
references allowed. Accepted papers will be part of the official ECCV proceedings.
[Download LaTex Template]
[OpenReview Submission Site] -
Extended abstracts not previously published or accepted for publication in substantially similar
form in any other peer-reviewed venues including journals, conferences or workshops. Papers are limited to
4 pages, and will NOT be included in official ECCV proceedings (non-archival), which are allowed for
re-submission to later conferences.
[Download LaTex Template]
[OpenReview Submission Site]
Accepted papers (full track).
- Paper ID 2: AnoVox: A Benchmark for Multimodal Anomaly Detection in Autonomous Driving - Daniel Bogdoll, Iramm Hamdard, Lukas Roessler, Felix Geisler, Muhammed Bayram, Felix Wang, Jan Imhof, Miguel de Campos, Anushervon Tabarov, Yitian Yang, Martin Gontscharow, Hanno Gottschalk, J. Marius Zoellner
- Paper ID 3: On Camera and LiDAR Positions in End-to-End Autonomous Driving - Malte Stelzer, Jan Pirklbauer, Jan Bickerdt, Volker Patricio Schomerus, Jan Piewek, Thorsten Bagdonat, Tim Fingscheidt
- Paper ID 4: ProGBA: Prompt Guided Bayesian Augmentation for Zero-shot Domain Adaptation - Jian Zou, Guanglei Yang, Tao Luo, Chun-Mei Feng, Wangmeng Zuo
- Paper ID 7: ReGentS: Real-World Safety-Critical Driving Scenario Generation Made Stable - Yuan Yin, Pegah KHAYATAN, Eloi Zablocki, Alexandre Boulch, Matthieu Cord
- Paper ID 8: Loop Mining Large-Scale Unlabeled Data for Corner Case Detection in Autonomous Driving - Jiawei Zhao, Yiting Duan, Jinming Su, yangwangwang, Tingyi Guo, Xingyue Chen, Junfeng Luo
- Paper ID 9: HumanSim: Human-Like Multi-Agent Novel Driving Simulation for Corner Case Generation - Lingfeng Zhou, Mohan Jiang, Dequan Wang
- Paper ID 11: Talk to Parallel LiDARs: A Human-LiDAR Interaction Method Based on 3D Visual Grounding - Yuhang Liu, Boyi Sun, Yishuo Wang, Jing Yang, Xingxia Wang, Fei-Yue Wang
- Paper ID 16: RoSA Dataset: Road construct zone Segmentation for Autonomous Driving - JINWOO KIM, Kyounghwan An, Donghwan Lee
- Paper ID 17: A Multimodal Hybrid Late-Cascade Fusion Network for Enhanced 3D Object Detection - Carlo Sgaravatti, Roberto Basla, Riccardo Pieroni, Matteo Corno, Sergio M. Savaresi, Luca Magri, Giacomo Boracchi
Accepted papers (abstract track).
- Paper ID 2: Challenge report: Track 2 of Multimodal Perception and Comprehension of Corner Cases in Autonomous Driving - Zhiying Du, Zhen Xing
- Paper ID 3: DreamForge: Motion-Aware Autoregressive Video Generation for Multi-View Driving Scenes - Jianbiao Mei, Yukai Ma, Xuemeng Yang, Licheng Wen, Tiantian Wei, Min Dou, Botian Shi, Yong Liu
- Paper ID 4: Two-Stage LVLM system: 1st Place Solution for ECCV 2024 Corner Case Scene Understanding Challenge - Ying Xue, Haiming Zhang, Yiyao Zhu, Wending Zhou, Shuguang Cui, Zhen Li
- Paper ID 5: NexusAD: Exploring the Nexus for Multimodal Perception and Comprehension of Corner Cases in Autonomous Driving - Mengjingcheng Mo, Jingxin Wang, Like Wang, Haosheng Chen, Changjun Gu, Jiaxu Leng, Xinbo Gao
- Paper ID 6: DiVE: DiT-based Video Generation with Enhanced Control - Junpeng Jiang, Gangyi Hong, Lijun Zhou, Enhui Ma, Hengtong Hu, xia zhou, Jie Xiang, Fan Liu, Kaicheng Yu, Haiyang Sun, Kun Zhan, Peng Jia, Miao Zhang
- Paper ID 7: From Regional to General: A Vision-Language Model-Based Framework for Corner Cases Comprehension in Autonomous Driving - XU HAN, Yehua Huang, SongTang, Xiaowen Chu
- Paper ID 8: A Lightweight Vision-Language Model Pipeline for Corner-Case Scene Understanding in Autonomous Driving - Ying Cheng, Min-Hung Chen, Shang-Hong Lai
- Paper ID 9: Iterative Finetuning VLM with Retrieval-augmented Synthetic Datasets Technical Reports for W-CODA Challenge Track-1 from Team OpenDriver - Zihao Wang, Xueyi Li
- Paper ID 11: FORTRESS: Feature Optimization and Robustness Techniques for 3D Object Detection Systems - Caixin Kang, Xinning Zhou, Chengyang Ying, Wentao Shang, Xingxing Wei, Yinpeng Dong, Hang Su
- Paper ID 12: Adversarial Policy Generation in Automated Parking - Alessandro Pighetti, Francesco Bellotti, Riccardo Berta, Andrea Cavallaro, Luca Lazzaroni, Changjae Oh
Important Dates (AoE Time, UTC-12)
Challenge Open to Public | June 15, 2024 |
Challenge Submission Deadline | Aug 15, 2024 11:59 PM |
Challenge Notification to Winner | Sep 1, 2024 |
Full Paper Submission Deadline | Aug 1, 2024 11:59 PM |
Full Paper Notification to Authors | Aug 10, 2024 |
Full Paper Camera Ready Deadline |
Sep 18, 2024 11:59 PM |
Abstract Paper Submission Deadline | Sep 1, 2024 11:59 PM |
Abstract Paper Notification to Authors | Sep 7, 2024 |
Abstract Paper Camera Ready Deadline | Sep 10, 2024 11:59 PM |
Schedule (Milano Time, UTC+2)
Opening Remarks and Welcome | 09:00 AM - 09:05 AM |
Invited Talk 1: Vision-based End-to-end Driving by Imitation Learning
Speaker: Antonio M. López |
09:05 AM - 09:35 AM |
Invited Talk 2: Reasoning Multi-Agent Behavioral Topology for Interactive Autonomous Driving
Speaker: Hongyang Li |
09:35 AM - 10:05 AM |
Invited Talk 3: Simulating and Benchmarking Self-Driving Cars
Speaker: Andreas Geiger |
10:05 AM - 10:35 AM |
Poster Session and Coffee Break | 10:35 AM - 11:05 AM |
Invited Talk 4: Long-tail Scenario Generation for Autonomous Driving with World Models
Speaker: Lorenzo Bertoni |
11:05 AM - 11:35 AM |
Industry Talk: Industrial Talk on Autonomous Driving
Speaker: Chufeng Tang |
11:35 AM - 12:05 AM |
Challenge Summary & Awards | 12:05 AM - 12:15 AM |
Oral Talk 1: Winners of Track 1 | 12:15 AM - 12:30 AM |
Oral Talk 2: Winners of Track 2 | 12:30 AM - 12:45 AM |
Summary & Future Plans | 12:45 AM - 13:00 PM |
Invited Speakers
Antonio M. López is a Professor at the Universitat Autònoma de Barcelona. He has a long trajectory carrying research at intersection of computer vision, simulation, machine learning, driver assistance, and autonomous driving. Antonio has been deeply involved in the creation of the SYNTHIA and UrbanSyn datasets and the CARLA open-source simulator, all created for democratizing autonomous driving research. Antonio’s team was pioneer on synth-to-real domain adaptation in the late 2010’s. Antonio’s team and colleagues also put the focus on vision-based end-to-end autonomous driving powered by deep imitation learning. Antonio is actively working hand-on-hand with industry partners to bring state-of-the-art techniques to the field of autonomous driving.
Hongyang Li is an Assistant Professor at University of Hong Kong and Research Scientist at OpenDriveLab, Shanghai AI Lab. His research focuses on self-driving and embodied AI. He led the end-to-end autonomous driving project, UniAD and won CVPR 2023 Best Paper Award. UniAD has a large impact both in academia and industry, including the recent rollout to customers by Tesla in FSD V12. He served as Area Chair for CVPR 2023, 2024, NeurIPS 2023 (Notable AC), 2024, ACM MM 2024, referee for Nature Communications. He will serve as Workshop Chair for CVPR 2026. He is the Working Group Chair for IEEE Standards under Vehicular Technology Society and Senior Member of IEEE.
Andreas Geiger is a Professor at the University of Tübingen and the Tübingen AI Center. Previously, he was a visiting professor at ETH Zürich and a group leader at Max Planck Institute for Intelligent Systems. He studied at KIT, EPFL and MIT, and received his PhD degree in 2013 from KIT. His research focuses on the intersection of computer vision, machine learning and robotics. His work has received the Longuet-Higgins Prize, the Mark Everingham Prize, the IEEE PAMI Young Investigator Award, the Heinz Maier Leibnitz Prize and the German Pattern Recognition Award. In 2013 and 2021 he received CVPR best paper and best paper runner-up awards. He also received the best paper award at GCPR 2015 and 3DV 2015 as well as the best student paper award at 3DV 2017. In 2019, he was awarded an ERC starting grant. He is an ELLIS fellow and coordinates the ELLIS PhD and PostDoc program. He maintains the KITTI and KITTI-360 benchmarks.
Lorenzo Bertoni is a Senior Applied Scientist at Wayve, where he works at the intersection of generative AI and autonomous driving. He earned his Ph.D. with distinction from EPFL in 2022, focusing on 3D perception for autonomous driving. His research emphasized improving safety for vulnerable road users and addressing corner cases. Lorenzo has published at leading computer vision and robotics conferences such as CVPR, ICCV, and ICRA. His work has been featured in EPFL news, various Swiss newspapers, and on the BBC 4Tech Show.
Chufeng Tang is now a researcher in IAS BU of Huawei. His research interests primarily lie in computer vision and autonomous driving. He received his Ph.D. degree from Tsinghua University in 2023, and B.E. degree from Huazhong University of Science and Technology in 2018.
Organizers
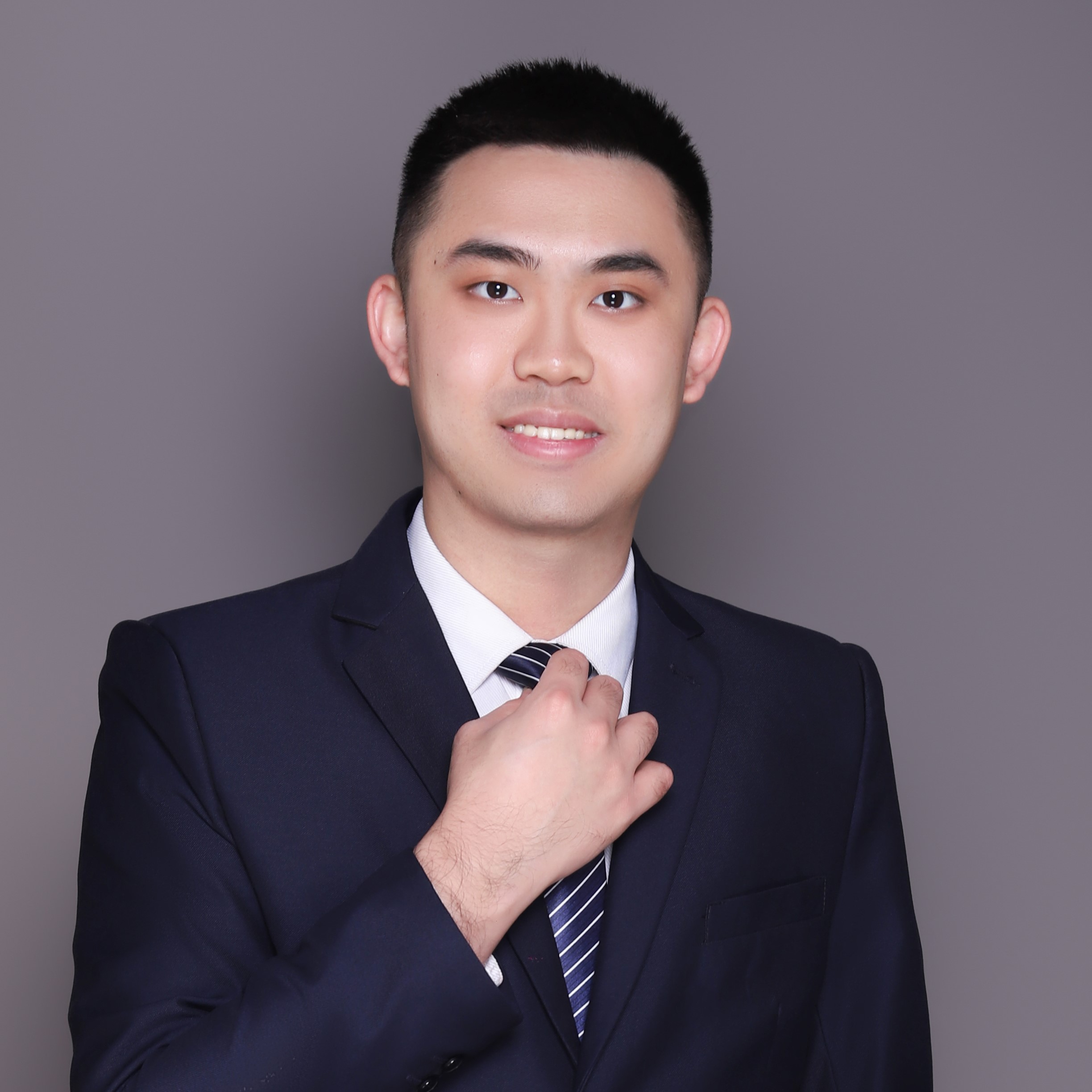
HKUST
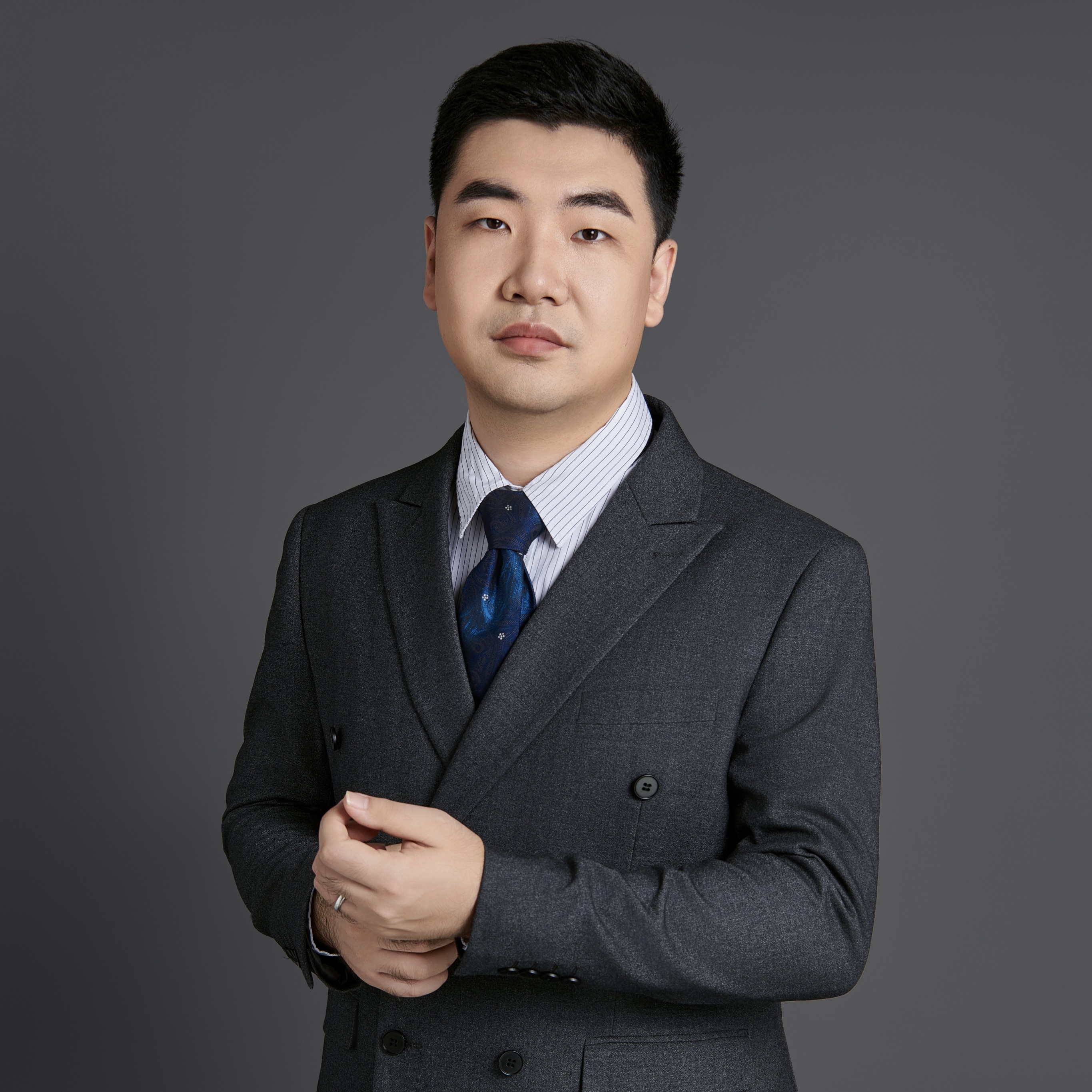
CUHK
Huawei
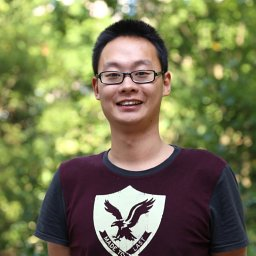
Huawei
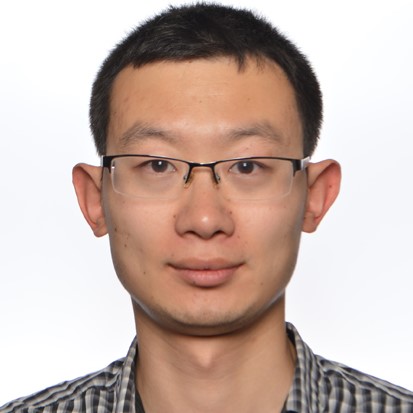
DUT
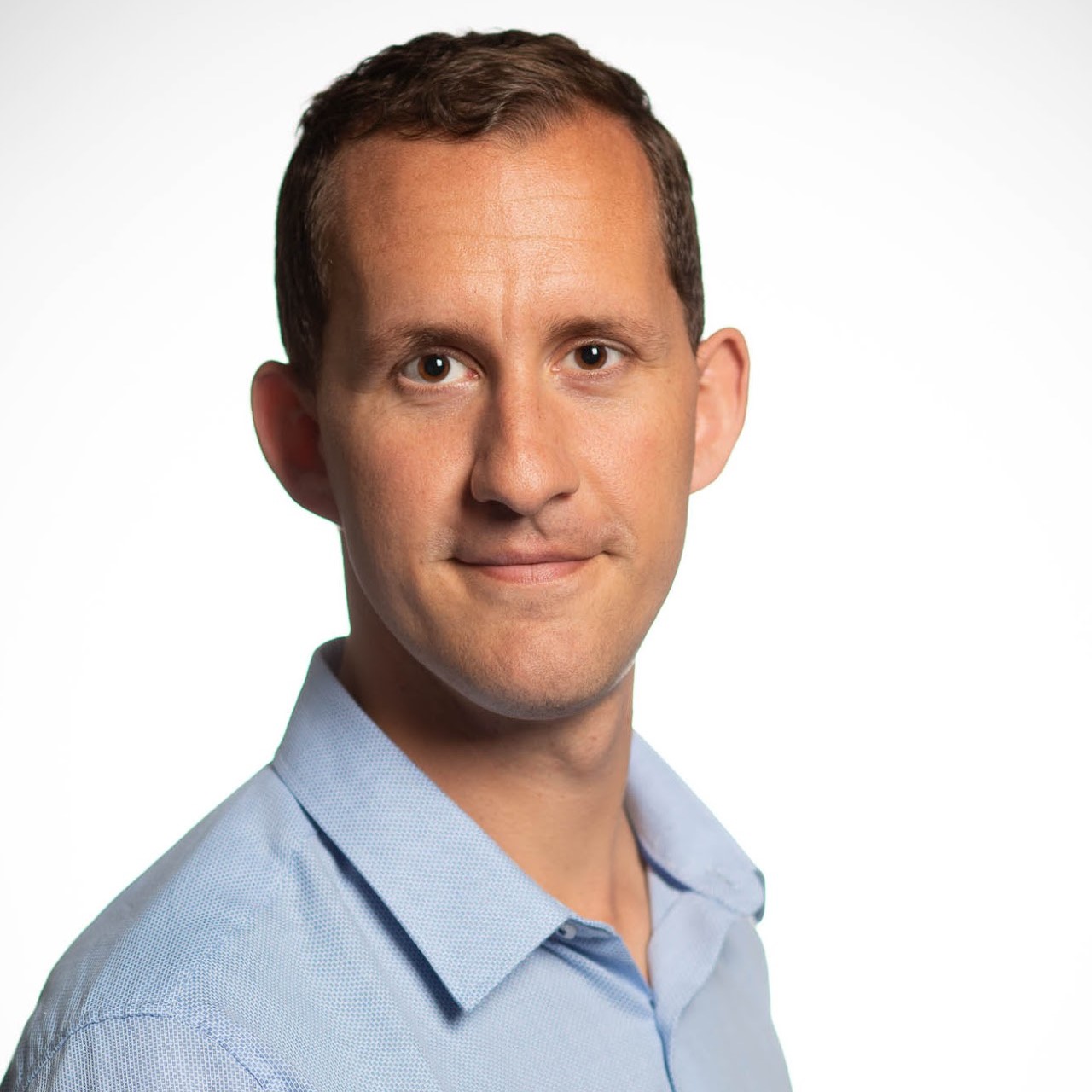
TU Delft
Huawei
Huawei
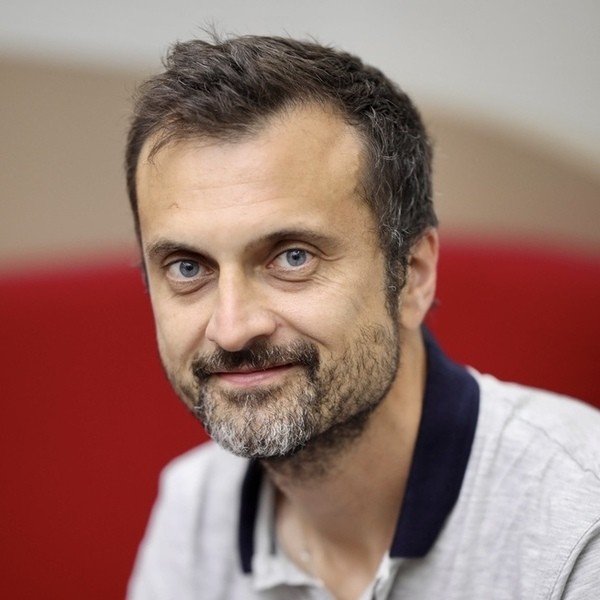
Huawei
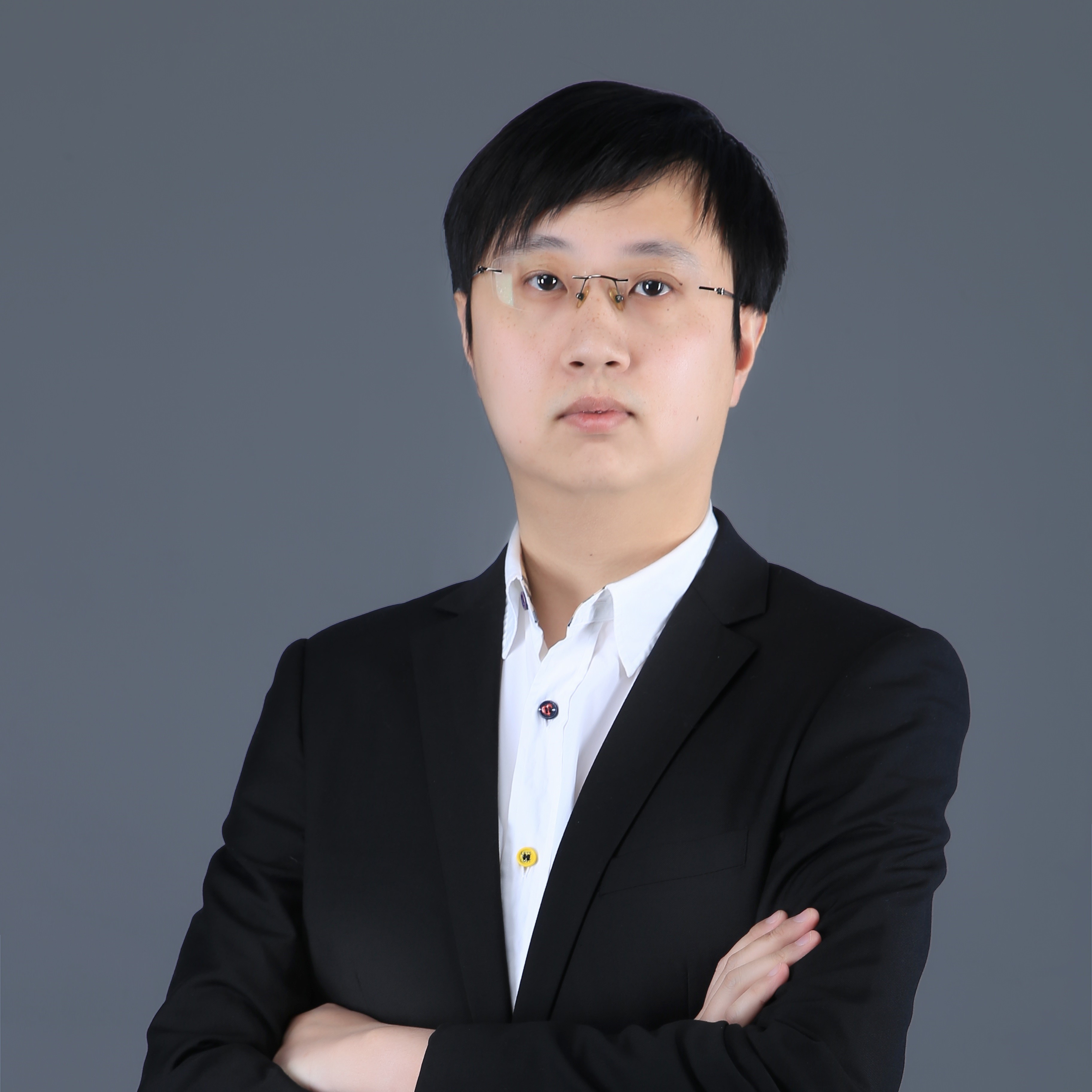
Huawei
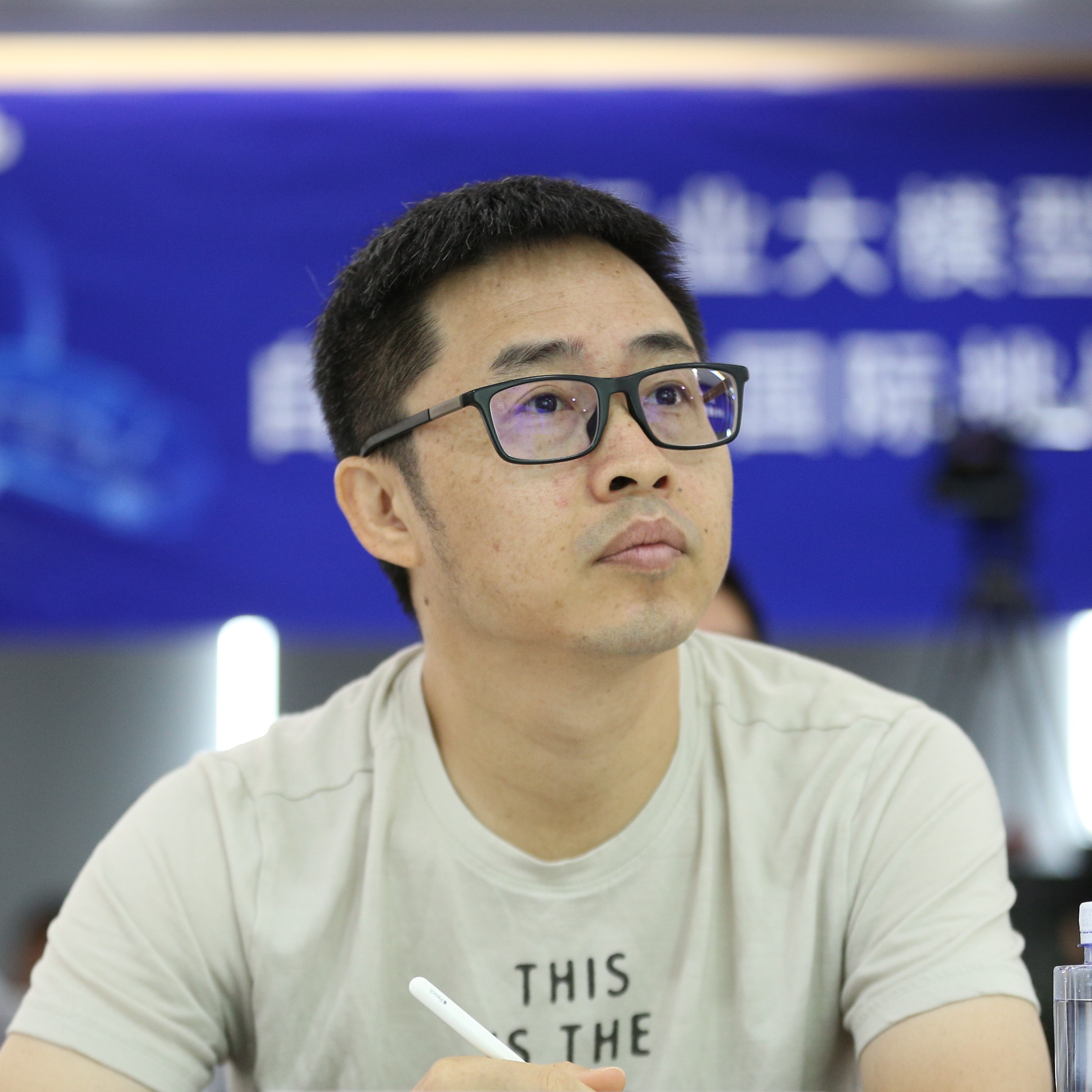
Huawei
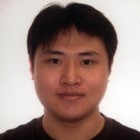
CUHK
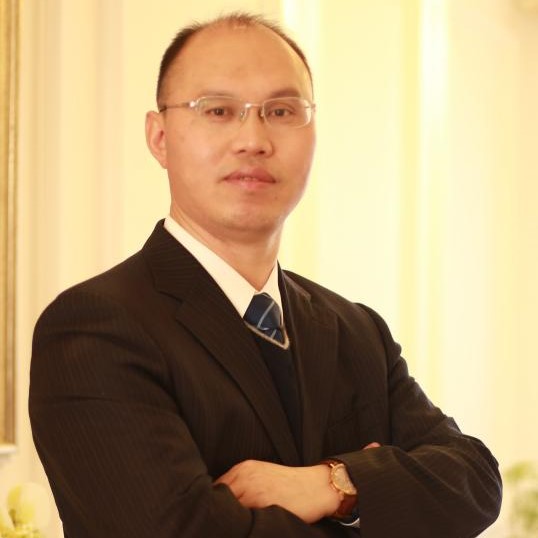
DUT
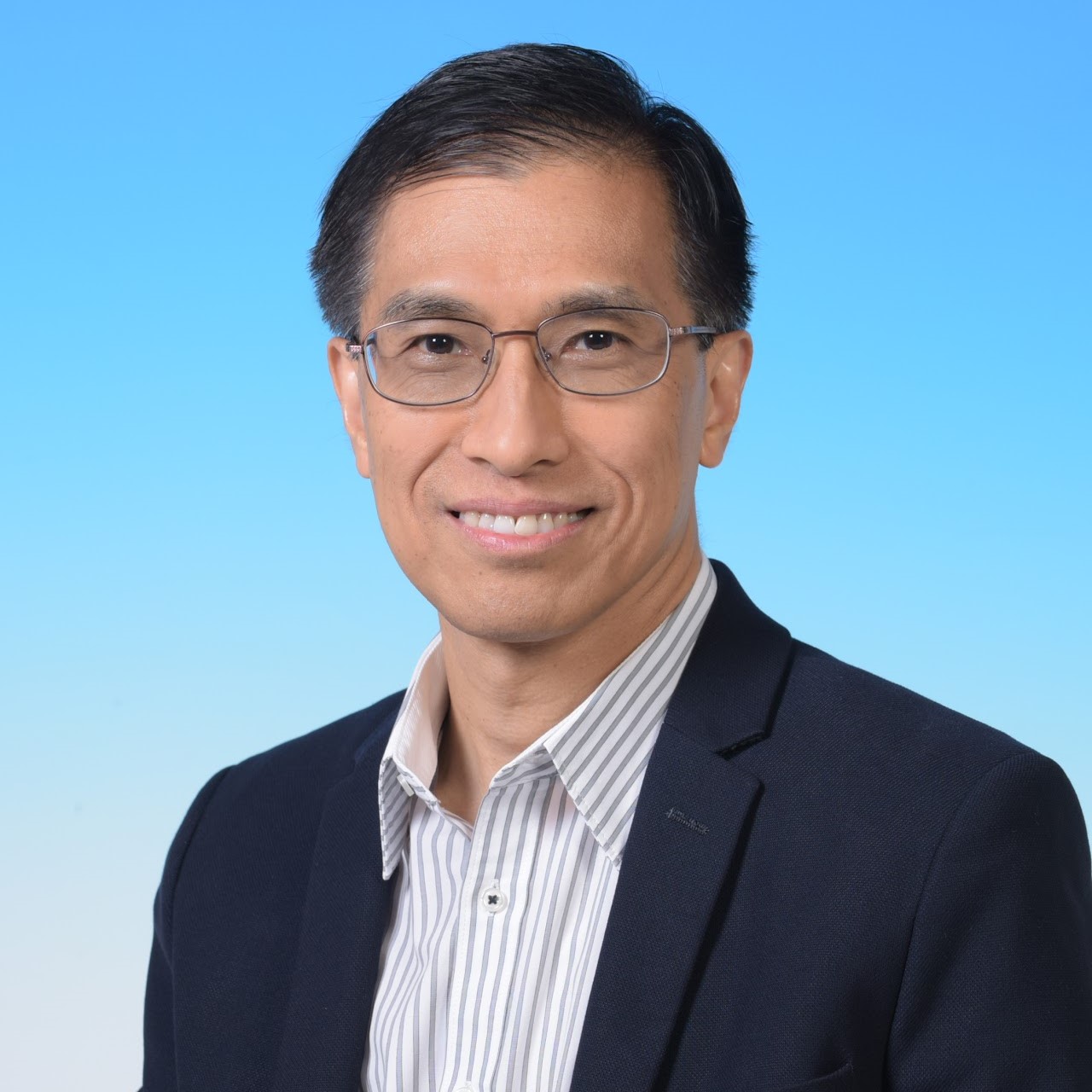